AGENTS OF SPATIAL INFLUENCE
CHAPTER I: DESIGN OF SPACE
Spaces like interiors and public locales are the environments we live in, and live with every day. It affects the way we perceive and behave. The way space is organized by interior elements, furniture, texture, color, and functional devices affect our perception and action. In terms of perception, the presence of windows in visual representations of space affects the social aesthetics and mood of the viewers (Kaye and Murray 1982). In terms of behaviors, the way offices are arranged can promote different levels of perseverant behavior by the way each room is partitioned (Roberts et al. 2019). Moreover, human capabilities like creativity and productivity are linked to how free or confined people feel within movable walls and furniture that support different sized groups (Taher 2008).
Studies have found faster reactions of participants in red-lighted rooms, which are more appropriate for active learning, as opposed to blue-green rooms, which may be suitable for passive participation (Drew 1971). People prefer symmetric room arrangement, while creatives like complex versions of the symmetry. Even more subtle properties like the temperature of rooms can affect aggression.
One of the most direct ways to study how space affects us to use different seating arrangements. In one study, subjects were asked to evaluate individual-oriented vs. family-oriented vacation advertising. The way subjects sat around a room in an angular or a circular seating arrangement imperceptibly affected how persuasive these different endorsements became (Figure 1). Those sitting at an angle were more likely to perceive the individual-targeting ads positively, while those sitting in a circle found the family-targeting material more acceptable (Zhu and Argo 2013). Other work finds that corner-table seating arrangements produce greater subject interaction than opposite-facing and side-by-side facing seating, and that subjects will choose rounded corner seats for purposes of discussion over other configurations (Sommer 1959). Therefore the way seats are arranged in a room affects the way participants perceive the scene and think about possible space of interactions within the scene.
If that’s the case, do interior elements affect perception to the extent that it affects intention? In a study involving social dining, it was shown that monochromatic lighting and dim lighting each affects the way people psychologically assessed the atmosphere, but only in the case of dining with “a special friend” (i.e. romantic diners) (Wardono, Hibino, and Koyama 2012). This means that certain environmental variables are especially considered in certain types of interactions, making the interior elements context specific.
If indeed interior design affects perception and intention, can it change human behavior? In a classroom experimental study, rearrangement of room furniture led students to move to different areas of the class, and different types of behaviors emerged (Weinstein 1977). Without using new furniture, the scientists changed the distribution of students in different areas over time and the tools (games, scientific instruments) they used most often simply by moving the furniture once. In the classroom context, one study calls for furniture to shift from functional to aesthetic appeal, and for high ceilings and fewer walls to promote flexibility and mobility (Warner and Myers 2010). One characteristic of creative environments involves movable benches, containers of tools, and cabinets that can be shared and adapted for use by multiple parties. There has also been a use of music to affect mood and decorative cues for cognitive stimulation.
By studying the way people gaze at the two different landmarks of TV and table in a scene, we can infer implicit human objectives when they view the scene. The TV screen is associated with paying attention to a common source of interest, while the coffee table is associated with discussions and social activities. If the arrangements of chairs can modulate these differing attention-inferred objectives, the chairs would serve as a source for possible implicit interactions with humans that influence their behavior by the way they are positioned (Ju 2015). By understanding the geometric cues that can influence human attentional and behavioral processes, chairs may serve as machines that modulate human interactions without human conscious intervention.
Considering this, I decided to study the way chair arrangements affect the way people pay attention to elements in a scene that lead to stereotypic behavior in a room, such as a TV screen for showing movies or coffee table for discussions. In addition, I created an immersive environment to test how arrangements affect interaction in hypothetical environments where chair configurations and interactions shape how and where people pay attention. By changing chair placement, we can affect what people do and where they go in a VR space.
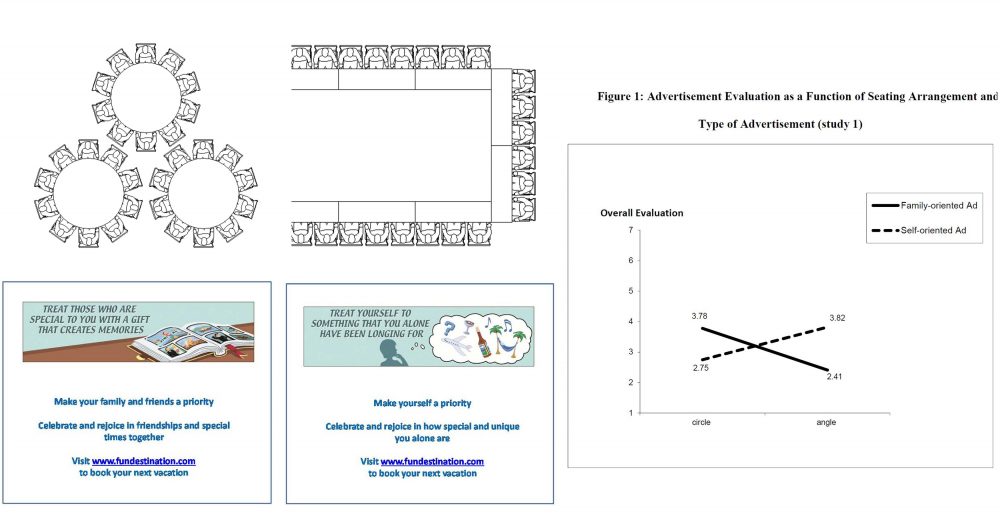
CHAPTER II: MACHINE GESTURES
The first way we communicate with others is with nonverbal cues like posture, facial expression, and gestures. Communicating with machines like user interfaces and robots rely on some of the same metaphors we apply to humans, such as “whether the system understand me,” “where is it telling me to go,” “what is going to happen now,” etc. The way we perceive these machine behaviors in human terms allows us to influence human perception and action by designing effective, relatable machine gestures. Effective communication with machines require an understanding of how humans interpret gestures and nonverbal cues of machines.
Studies of gestures in human-machine communication have focused on understanding situations when humans use gestures in different contexts to communicate to different real or artificial agents (Mol et al. 2007), and in designing artificial systems that detect and respond to human gestures. When people were given the opportunity to evaluate the gestures of robotic agents, a study found that they prefer gestures most like their own (Luo, Ng-Thow-Hing, and Neff 2013). This is analogous to unconscious mimicking of gestures during human-human conversations.
My previous work has also examined which robotic lamp and micro-machine gestures elicit human compassion and understanding by creating a story of a troupe of robots which perform when the audience is not looking (LC 2019). This work begins to probe the type of robotic furniture gestures that are best at eliciting human empathy, gestures like turning away in shyness and up-down movements for agreement (Figure 2). This work is related to studies by other labs with a mechanical ottoman footstool, where a furniture robot was able to get people to rest their feet on it, as well as understand a cue for getting the feet off as an up and down gesture (Sirkin et al. 2015). A chair robot was able to get people to move out of the way after using overt gestures like moving forward-backward or side-by-side while it moved across the room (Knight et al. 2017). In that experiment, different physical chair gestures communicated the idea that the human subject should move out of the way so it can get to the cabinet. Of the three gestures tried (shaking left and right, subtle pause, and moving back and forth), the one that is best able to get humans to move for the chair is the back and forth movement, which best suggests that the chair is in the business of moving past the person to the cabinet (and is trying to step forward). This suggests that bystanders best respond to clear communication strategies from object furniture and don’t want to be overly interrupted. Together these robotic furniture studies show that robots can communicate with humans with overt gestures based on movement, and that humans will perceive them with different efficacy depending on context and motivation. Furniture that interpret human response and use gestures to communicate will make environments more amenable to bidirectional communication with humans, and to influence them to respond in positive ways.
Considering this, I studied the way people perceive gestures performed by chairs. In order to see which particular interactions are perceived to be human-like and sensible, I worked with a team at Cornell Tech to qualitatively code human responses to videos of human-chair interactions to separate out potential gesture sets. I gleaned the insights from the gesture study to design explicit movements and interactions in a VR environment to see how people react to animated gestures in space. I found that communicative actions in VR can be represented by physically realistic but hypothetical machine gestures that test possible interactions without having to build the machines themselves.
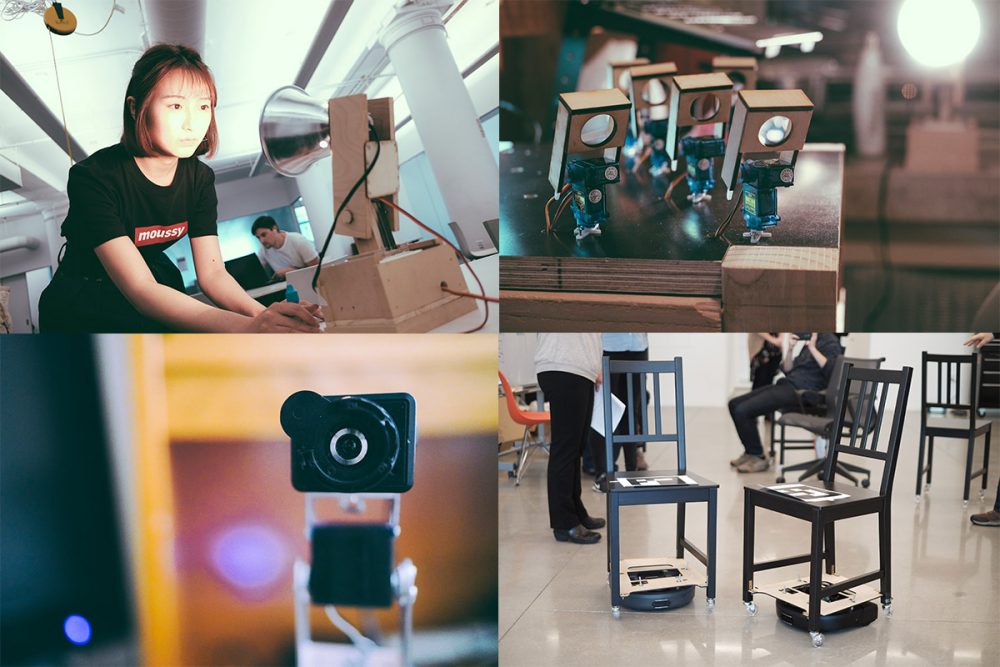
CHAPTER III: DESIGN FOR SOCIAL INFLUENCE
Knowing the way human perceptions and actions are shaped by the space they inhabit and the gestural interactions of machines they communicate with, can we design for positive social influence by drawing from a palette of effective design patterns that situate machines and their actions in specific contexts?
One approach coined the term “Mindless Computing,” referring to use of persuasive technology on a subconscious level to do good (Adams et al. 2015). The study describes an approach of sending System 1 (automatic, subconscious processing system) output to System 2 (decision-making, conscious processing) in a network for human behavioral change. The principle of “least effort” is regarded as using heuristics to save effort so that we can “nudge” humans to better behavior. In comparison with System 2 strategies like goal-setting and self-monitoring, System 1 influence strategies don’t rely on human motivation and self-control (which are difficult to control) but rather rely on “non-interrupting” reflexive interventions that run in parallel to other cognitive systems to trigger behaviors much as in daily life so as to not burden the user to too much information. The study then gives two example projects utilizing these strategies: 1. a technology that changes the color of the inner plate to match the color of food to make the food appear more than it really is, 2. a voice matching method that lowers the pitch of the voice of the feedback to get users to lower the pitch of their voices. The former technology gets people to eat less for health; the latter method gets people to have a deeper, more confident voice.
Perhaps one way machines can exert their influence is in collaborate with humans. One study described the Human Robotic Interaction (HRI) paradigm based on perception of humans, verbal and nonverbal communication, affect and emotion, as well as higher level competencies like intentional action, collaboration, navigation, and learning (Thomaz, Hoffman, and Cakmak 2016). In discussing robot planning of navigation, they introduced the Social Force Model for robot navigation given an avoidance map to avoid humans or obstacles, and sensor data. Robot and human navigation is a two-way process, however, as the “freezing robot” phenomenon of robot planning in a crowded area shows. Another problem is to combine navigation to landmarks with human verbal directions to negotiate the totality of what the robot has to respond to. The intention of humans in HRI is investigated in a work that uses a theory of mind kept track of by the robot to infer the human’s plan and whether the human needs help (Görür et al. 2017). The robot and human make collaborative decisions, with the robot calculating a probabilistic model of the human’s intention, making its actions less intrusive and more appropriate.
Data-driven approaches have also been used in situating machines in particular spatial contexts. By comparing data-driven robot behavior generation vs. human heuristic planning in a “Mars Escape” game with another player, a study showed that in-the-moment interpersonal dynamics greatly affects interaction quality (Breazeal et al. 2013). The game models a search-and-retrieval task with a human astronaut and a robot on Mars. It is designed such that collaboration and communication is necessary to complete the mission. The real world game mimics the virtual game with museum visitors, using a robot to execute the planning tasks like “go to barrel,” “pick up canister,” “go to center.” Collaborative tasks emerged when least expected, and the amount of manual override by the human was minimized. When humans bid the robot to do something, there were often moments where the robot turned away or failed to acknowledge. This was when the interaction can turn south, causing an emotional disconnect. Humans simply want interpersonal recognition from such a bid.
The research detailed so far takes place almost exclusively in the lab, but people interact with machines in complicated social settings. One group calls for HRI labs to go to workplaces, homes, and public arenas to study the complex dynamics involved when robots are asked to work with multiple people (Jung and Hinds 2018). They argue for an understanding of robots in imperceptible contexts where their involvement follows complex rules of social interaction inherent in the public domain.
These considerations of how machines in particular spaces can shape human behavior suggests that a framework for designing human-machine interactions based on both spatial and gestural influence. Our goal will be to analyze which particular patterns in design of future smart contextual machines serve specific goals in different situations by examining human response to both contextual and gestural cues.
CHAPTER IV: FRAMEWORK
To bring both implicit and explicit influences on human perception and action into one theoretical underpinning, I propose the Environment Support Model for human-robot-environment interaction (Figure 3). I study chair robots because they sit at an intersection between being arranged in space, and also endowed with gestural capabilities using locomotion and movement. Some furniture like tables or sofas can be used to divide space for spatial influence but cannot be easily moved using motors to elicit machine gestures. Other devices like robotic arms can give intimate and precise gestures but do not affect spatial perception easily the way chairs can arrange themselves in a room.
The model suggests that humans interact with machines with gestural capabilities (like the lamp, surveillance camera, etc.) interactively and with explicit nonverbal cues, but that the agent aligns with the environment to shape human behavior subconsciously using space design that affect humans implicitly via arrangement (like furniture placement, space separation). In the context of chair robots, they serve both as agents with gestures that interact with humans directly, and be the effector of environmental influence by arranging themselves in a room with little attention (e.g. when people are not looking). Chair robots bring both wings of the model together to serve as agents with both explicit interactions and ability to influence spatially. Design for these agents are informed by human-machine sociology.
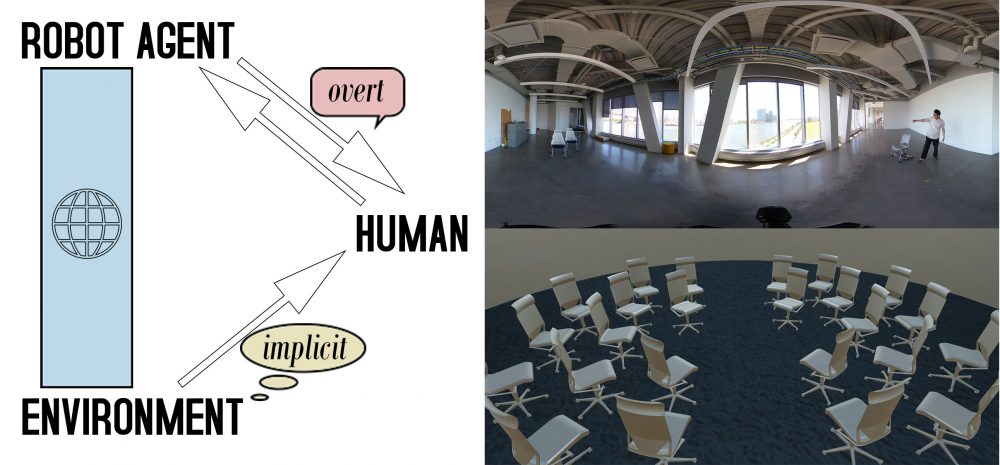
CHAPTER V: A STUDY OF SPATIAL INFLUENCE
To investigate how space affects human perceptual attention (the environment to human connection in the HRI Environment Support Model), I began with an experiment utilizing eye-tracking to gauge human visual attention in a scene, and see how different arrangements of chairs in that scene affects where people are gazing.
I hypothesized that when chairs are configured in alignment towards a common screen at the back of the room, people will tend to look at the screen in that scene, but when chairs are arranged in a circle around the coffee table in that same scene, more gaze time will be spent on the table. The screen and the table serve as symbolic areas of interest (AOI) that signal to us what the purpose of the room would be: a place that shows a movie or stages a lecture vs. a room for human socialization and discussion. The results show that attentional gaze towards objects associated with human activities (TV for watching and table for discussing) is influenced by the arrangement of furniture that enable such activities. This suggests ways to influence human behavior by adjusting the configuration of furniture in a room.
Methodology
I first created photos of the same view of a room containing a TV and a table but with different arrangements of the same chair, with the view of capturing the variabilities relevant to gaze behavior in a room with a TV and a coffee table. Previous study showed that variance in human evaluations of physical properties of classrooms can be explained by the three variables of view of outdoors, comfort, and seating arrangement (Douglas and Gifford 2001). Thus in addition to varying the configuration of the chairs in either facing the same direction or in a circle, I varied the presence of window vs. solid wall, and the density of chairs in the room.
In addition, I created additional conditions of having different colored TVs (that are on) and different colored tables. The idea is that they would emphasize those particular objects in the scene, serving as a control way of directing gaze attention to them. Finally, I added an intermediate condition between total alignment and totally circular arrangement called the semicircular arrangement. I reasoned that such a configuration should support both gaze to the TV and the table, serving as an intermediary control for the aligned and circular conditions. All renderings were done from the same camera location on a Unity scene with assets from the Vertex Studio furniture pack. The TV and table were measured to have the same screen area.
Experiments were run using IMotions 7.0 software to track gaze using a Tobii X2 30Hz eye tracker and facial expressions using a webcam and the Affectiva Affdex SDK. 8 students (2 female) from Northeastern University College of Art, Media, and Design participated in the study (Figure 4). Each subject was asked to fixate on a cross before presentation of each stimulus image, and to subjectively score on a scale of 1 to 5 how pleasant the scene depicted is in their mind. This was to make sure they are not consciously controlling the gaze, but is rather involved in some unrelated task while their eyes wander around the scene. 15 stimuli were each presented for 5 seconds after a 5 second fixation cross period for each image (Figure 5). The stimuli groups were Chair Aligned, Chair Circular, and Chair Semi-Circular conditions, each of which include the Normal Stimulus, Emphasize Table, Emphasize Chair, No Window, and High Density conditions. Participants were given each of the 15 stimuli in random order, given a rest period of 3-5 minutes, then presented with a reversed order of the stimuli, with the two result averaged.
In the eye-tracking analysis, the TV and table areas were drawn as Areas of Interest (AOI), and the time spent and percent time spent in the AOIs by the gaze points are calculated. Number of fixations in each condition in each AOI is also calculated, along with metrics like revisits (an indicator of confusion or sustained attention or frustration), ratio of participants who looked at the AOI, first and average fixation duration, and gaze heat maps. IMotions 7.0 software suite are used for the AOI marking and video of eye movements. The unprocessed results for each AOI in each condition are downloaded and analyzed in R 3.6.0.
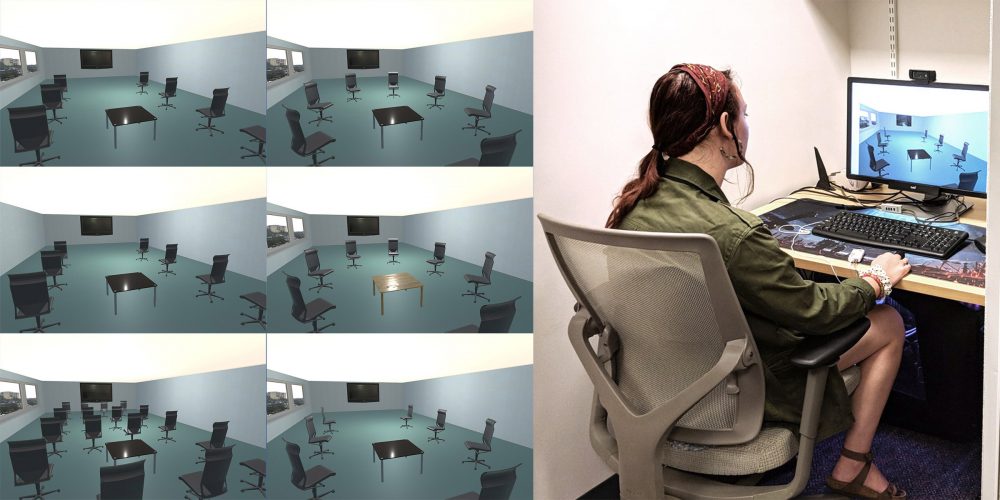
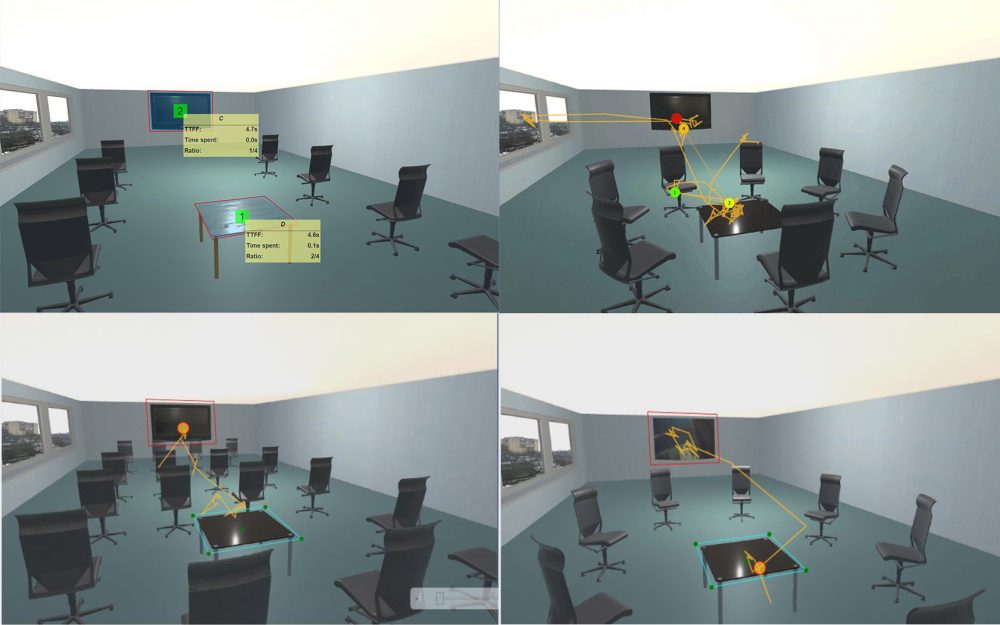
Results Summary
Examples of gaze heat maps of grouped data are shown in Figure 6, showing the concentration of gaze on the TV when chairs are aligned, and on the table when the chairs are arranged in a circle. To assess if the time spent gazing is affected by the location of the gaze (aoi factor) and the way chairs were placed in the scene (config factor), I performed a two-way ANOVA on data with the aligned, semi-circular, and circular config conditions. Results show only significant interaction aoi:config (F=6.698, df=2, p=0.00299), and no significance on aoi nor config by itself (Figure 7). The interaction plot shows that the biggest differences come when the chairs are aligned, and the participant’s gaze towards the TV is 15% more likely when the chairs are aligned than when they are circularly arranged (lower left). The trend is reversed for the table, making the interaction significant.
Next I examined the regular condition data that includes the semi-circular config, and found that again only aoi:config interaction is significant (F=6.698, df=2, p=0.00299). The semi-circular config led to about equal amount of time spent between TV and table conditions, fashioning itself as a control level between aligned and circular configs (Figure 7). Semi circular config also serves as a control for chairs facing the AOIs. In both aligned and circular conditions, chairs face the AOI with greater percent time spent, but in semi circular config, the chairs as a group face both the TV and the table AOIs, in effect removing that bias.
Posthoc comparison (Tukey) reveals a significant difference between tv:aligned vs. table:aligned (p=0.0040745), showing that subjects are more likely to look at the TV if the chairs in two rows are facing the wall containing the TV. The tv:circular vs. tv:aligned comparison is also significant (p=0.0045019), showing a difference in the way gaze is directed towards the TV when chairs are arranged in a circle around the table as opposed to aligned towards the wall. The table:semi vs. tv:aligned (p=0.0118714) and tv:semi vs. tv:aligned (p=0.0172216) are also significant, showing that the bias for TV AOI in gaze in aligned config is not due only to the chairs facing the TV, for in the semi- circular condition the chairs also look at the TV from each side, but there’s no preference for gazing at the TV. Thus, it’s the alignment of the chairs (not the facing toward the AOI) that affected gaze.
My other analyses shows that interaction of TV:table AOI with aligned:circular chair config is not affected by whether windows are present in the scene, but that coloring the AOIs to make them more conspicuous for gaze has about the same attentional effect as the alignment of chairs for gaze to the TV. To see whether varying the density of the chairs affects percent time spent in gaze for particular AOIs and configs, I ran a 3-way ANOVA that shows that high density arrangements increase time spent gazing (F=6.657, df=1, p=0.0116), but that this affects TV and table AOIs similarly (Figure 7). This 3-way interaction aoi:config:density (F=3.473, df=2, p=0.0356) means the way gaze occurs in an AOI in different chair configs is modulated by the density/number of these chairs. Thus I ran separate two-way ANOVAs on the high and normal density data. In both cases there are significant interactions aoi:config (p=0.00299 in normal, p=0.00147 in high density), but the 3-way result suggests that the interactions are different in normal and high density. Indeed, while in normal density, greatest gaze time occurs with TV AOI in aligned condition; in high density, greatest gaze time occurs with table AOI in circular arrangement. This shows that the number and density of chairs in a scene can affect the strength of the interaction, and even shift the interaction to favor certain AOIs. I postulate that the proximity of the chairs to the table is a stronger determinant of gaze attention than simply stacking more chairs in alignment, because it makes the semantics of the implicit conversation/meeting goal stronger. When chair density is normal, most of the effect comes from gaze to the TV in aligned config, but when chair density is high, the interaction comes more from gazing at the table in circular arrangement. This suggests people are more affected by the social meaning of tables when there are more chairs.
Discussion
The way rooms are arranged subtly influence us, both in terms of what we look at, and also what we perceive to be the core function of an environment. This study shows that chair arrangement affects the attention of humans towards targets that symbolize particular functions of a room. In a room with both a TV and a table, chairs aligned all facing the wall the TV is on focused gaze to the TV, implying a perception of the context for presentation, or watching video. On the other hand, chairs arranged in a circle around the table shifted attentional gaze to the table, as perception shifts to discussion, meeting, and socialization. Further data showed the lack of effects on facial expression (as opposed to gaze) between different conditions, indicating that participants reacted similarly to different configurations, and differences in gaze are not attributable to emotional and engagement factors during the course of the trials.
In relation to the Environment Support Model (Figure 3), I’ve shown that the environment can be utilized by artificial agents to make subtle changes that shape how humans perceive and act, giving possibilities for leveraging persuasive technologies to nudge humans to do good in specific contexts. Since human perception of what spaces are for and what interactions are possible are colored by what they see in the environment, robotic modification of these subtle environmental perceptions can change human behavior. The way space is arranged by chairs can influence us, both in terms of what we look at, and also what we perceive to be the function of an environment.
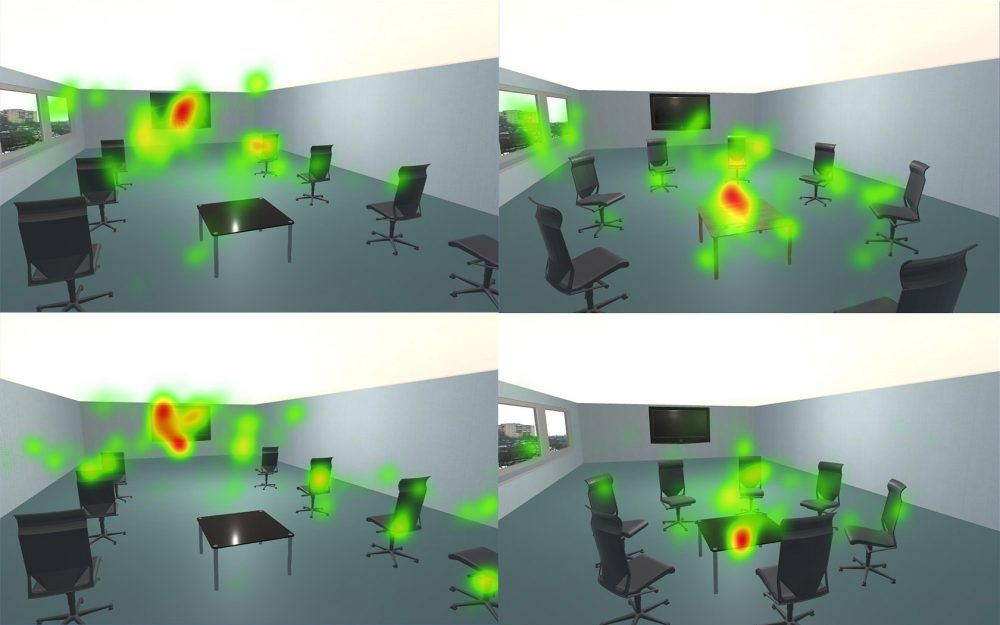
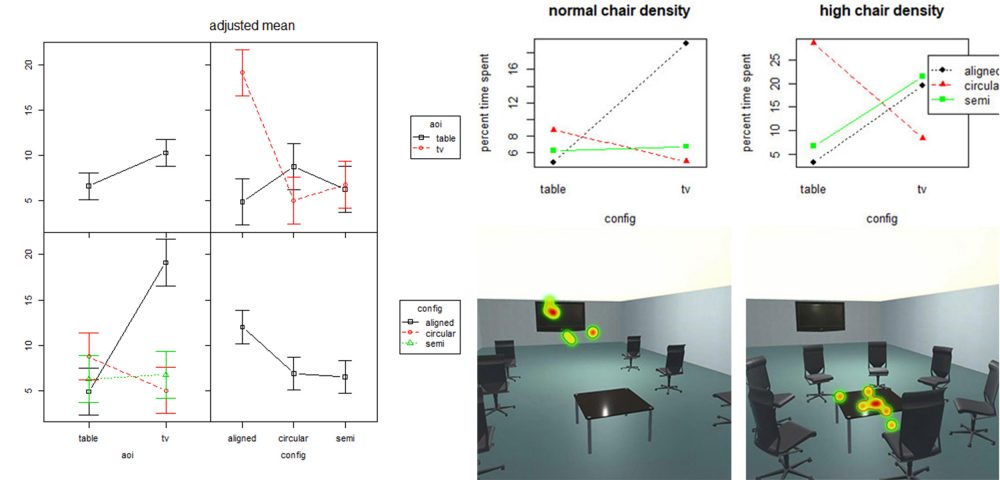
CHAPTER VI: A STUDY OF AGENT GESTURES
While spatial arrangement is important for perceptual influence, the role of gestures by autonomous agents also shapes human emotional response. To investigate which particular overt gestures undertaken by chair robots best communicate to human about goals and intentions of locomotive agents (the robot agent to human connection in the HRI Environment Support Model), I worked with a team at Cornell Tech to create videos of human-chair interactions and used crowd-sourced human surveys to see how people perceive different chair gestures.
The expressive relationship of the chairs to the actors should depend on the chair both being an agent of change and being an agent receptive of change. The chair has a relationship with the actors such that it can show understanding, disagreement, and responsiveness, while also being able to perform actions that communicate meaning. Therefore I hypothesize that the perceived most expressive chair gestures will also show a high rating of the chair being responsive to the person and the chair having a great effect on the person.
Methodology
I used a set of gestures that involved one or more persons interacting with one chair that has agency, or with multiple chairs that work with or against each other. Videos of one-to-one gestures of communication are based on interactions originally made by Natalie Friedman and Wendy Ju of Cornell Tech. As reformulated here, they include “Follow Me” where the chair moves forward in space in the direction it wants the person to go, “I Understand” where the chair moves back and forth to signal acknowledgment, “I am Not Going” where the chair shakes left and right to indicate it can’t move, “I am Occupied” where the chair shakes so that the human can take a different seat, and “I am Available” where the chair slides behind the human to show that it can be sat on. Other one-to-one gestures deal with human-chair co-locomotion, including “I am Tracking You” where the chair rotates in the direction of the human walking, “After You” where the chair lets the human go first when they encounter each other by stopping, “I Avoid Collisions” where the chair walks around the preoccupied human (on a cell phone) in a case where she is not cognizant of the interaction, and “I am on a Mission” where the chair is the one not stopping for anyone as it locomotes (Figure 8).
I further designed a set of interactions based on scenarios of multiple chairs helping to promotes certain types of activities in multiple people. This includes “Work Together” where two chairs arrange themselves opposite each other to promote people talking to one another, “Sit on Me Please” where two chairs compete to see where one human will choose to sit, “Let’s Help Out” where one chair comes into a situation with two humans and only one seat and offers itself to one of the persons, “Let’s Focus” where the chairs arrange themselves aligned looking forward (as in the aligned config in the Spatial Influence study) so that two humans can sit on them and focus on material on the wall, “Let’s Walk Together” where two chairs align themselves in speed so that the two humans following them can engage in chatting, “Stop Moving” where two chairs bind the moving human so that she cannot go forward or backward in space, and “Let’s Go” where a chair pushes for a human to get up from a different chair and start moving (Figure 9). These gestures are all directed at influencing human behavior and how they cooperate by using intrinsic chair-based interactions like locomotion and setting up particular arrangements.
Next I made green-screen recordings of the interactions with student actors. One actor wore a green suit in order to play the role of the chairs making gestures, while two actors served as human participants. A chroma key is used to convert green-screen content to a background image of the wall in Adobe Premiere, and the results are cropped to the same size.
The videos are shown to human workers on Amazon Mechanical Turk. Participants are asked to rate for each video under the following criteria: “Chair was responsive to the person” (Responsive), “Relationship between chair and the person in the scene is satisfactory” (Relationship), “This chair is very expressive” (Expressive), and “The person in the scene is affected by the chair’s presence” (Affected). The first question examines perception of the chair’s reaction to the person’s actions, while the last question studies perception of the person’s reaction to the chair’s actions. In addition to the ratings, qualitative questions like “Describe what you saw,” “What’s the chair’s intent?”, and “What is the chair communicating to the person?” are also given to the workers and the answers are coded by two independent raters whose correspondence is tested using the Cohen’s kappa metric.
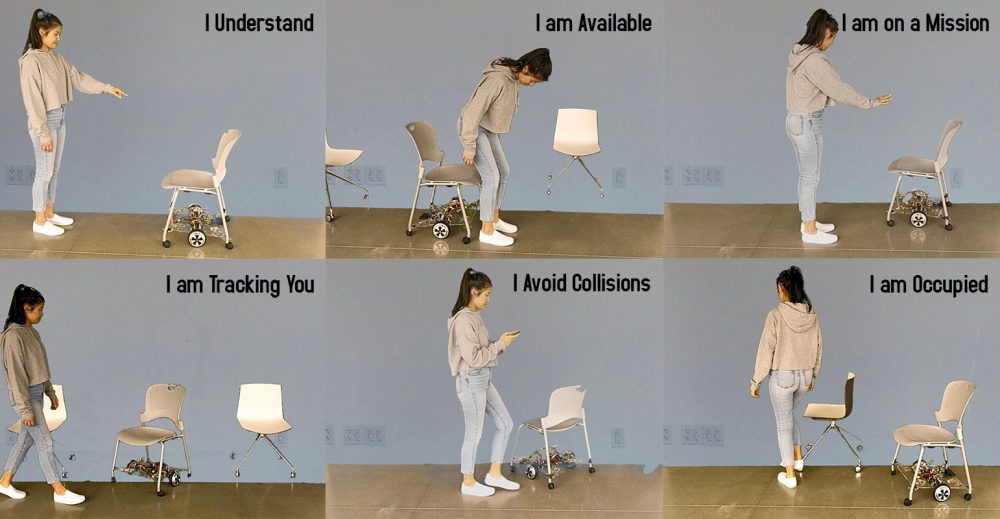
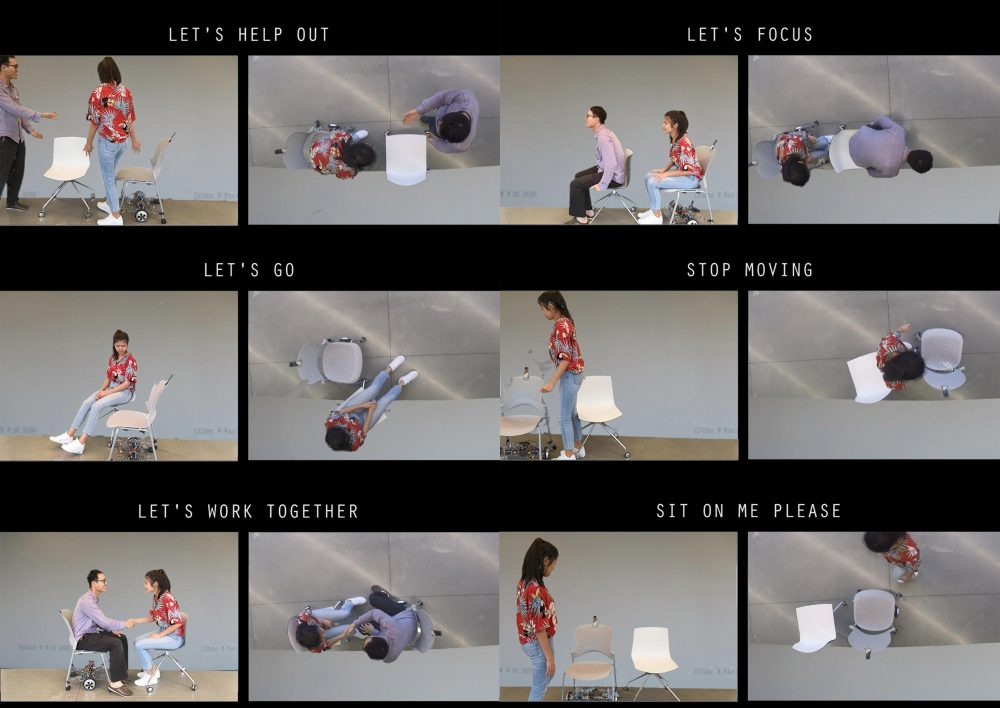
Results Summary
To see how ratings vary with the question asked and the interaction video showed, I ran a two-way ANOVA using Interaction*Question as explanatory variables, and found Interaction, Question, and Interaction:Question to be significant (p < 0.05). Posthoc test (Tukey) reveals Expressive-Affected, Responsive-Expressive, and Responsive-Relationship to be significantly different, but that Responsive and Affected ratings are not significantly different (p=0.6014), indicating that whether chair serves as agent of change or subject of change by the person does not affect ratings about its expressiveness and relationship with the person.
Summary data (Figure 10) shows that “I am on a Mission” scored the lowest in all questions except Affected, because the chair acts independent of the person in going its own way. Interactions with high ratings on Affected tends to have high Responsive scores also, as suggested by the posthoc comparison (e.g. “I am Not Going,” “Stop Moving,” and “Let’s Go.”) “I Avoid Collisions,” “I am Tracking You,” and “After You” all scored low on Affected because the person goes her own way without being interrupted by the chair in both cases. The proportion of variance attributable to Interaction (eta η2) is 0.15, to Question is 0.027, and to Interaction:Question is 0.23, showing the different responses to each question based on the video.
To see how each of the questions correlate with each other on each video of interaction, I ran a multiple regression model using Interaction*Question as explanatory variables (Multiple R2=0.4004). Only the coefficients for the Relationship and Responsive questions are significant, suggesting that the other variables may be correlated with these two, so that regression only need these two to explain the ratings. If I ran separate linear models for the ratings to each separate question, I get a similar result, where for the Responsive and Relationship data, the R2=0.4617, and 0.4243, respectively, but for the Expressive data, R2=0.2002 (Figure 10).
To verify this finding, I computed the correlation coefficients between data from Responsive and Expressive (0.5657), Relationship and Expressive (0.1502), and Affected and Expressive (0.2239). This shows that Expressive is not a great explainer for the ratings because it correlates with Responsive, which explains a great deal of variance in the data. This lends support to one part of the hypothesis that chairs that appear to be responsive to the person in the video is perceived as expressive, even though its behavior is in reaction to the person.
Discussion
This study shows that people’s perception of whether chair expressions are affecting humans is linked to whether chairs are responsive to humans. This underlies the idea that chairs and humans affect each other in feedback loops, so that agency from one side is reflected in agency in the other, as they mutually influence each other. Both sides of the influence are reflected in the question of expressivity, which summarizes the interactions amongst chairs and people.
The methodology utilized by our team at Cornell Tech allows us to evaluate which robotic gestures are effective in communicating intent and response to humans using crowd-sourced data evaluation. For example, “Let’s Go,” “I am Occupied,” and “Stop Moving” are most effective at evoking perception that the chair affects people by performing the gesture. On the other hand, chairs in “Follow Me,” “I Understand,” and “Let’s Help Out” are best perceived as being responsive to the human. In particular, “I am Available” and “Let’s Help Out” score high on both responsiveness and ability to affect, giving them a perceived high level of relationship with humans in the scene. I also noticed that the “I am Tracking You” gestures is perceived to be highly responsive to the actor while not affecting her behavior at all (Figure 10). This means that the Tracking You gesture itself does not do anything that would change the way participants behave, a useful property that ensures its inclusion in virtual environments can be used to study purely perceptive effects of participants in the scene. This video prototyping strategy allows us to plan and posit certain chair gestures as maximally effective at evoking particular responses from humans.
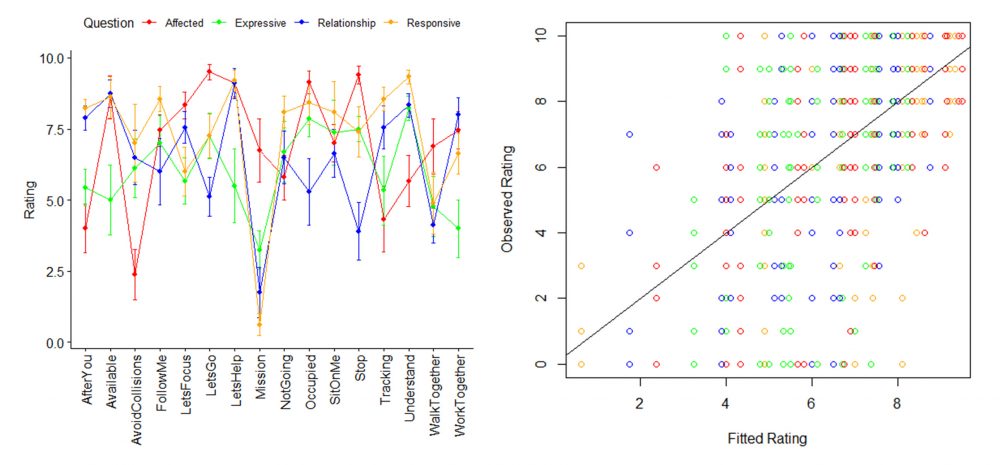
CHAPTER VII: A STUDY USING 3D IMMSERSIVE PROTOTYPES
I have shown that arrangement of locomotive agents in space affects perceptual attention and people’s understanding of possible interactions in a space. I have also shown that particular overt gestures of these locomotive agents (chair robots) are best able to evoke perceptions of chair responsiveness or ability to influence human action. These two results begs the question of whether we can create prototypes of chairs in space that both rearrange themselves to affect us implicitly, and also make gestures that communicate with us their intentions directly. Since the power of spatial design relies in having many chairs all arranged in a particular configuration, can we prototype these interactions without having to build all these chairs with proper mechanical controls?
Instead of only measuring attention as in 2D screen-based prototypes, and of only evaluating audience perception as in the video-based prototypes, I endeavored to model interactions in VR space with virtual chairs that may do things that they don’t do in real life, in order to evaluate the effectiveness of particular interactive gestures in different spatial configurations, and study the way audiences gaze in that space and interact with objects in locomotion. One test we can make in this virtual space is to see how audiences are affected by the way chairs behave in particular arrangements. I hypothesize that chair gestures in particular configurations evoke greater audience response.
Methodology
I created a set of possible chair arrangements in VR and ended up picking two for further analysis, the fully aligned and circularly assigned configurations that are reminiscent of Spatial Influence study (Figure 11). Instead of simply evaluating perception and attention, VR allows us to examine interactions with virtual prototypes that react to us in space and time. I chose to focus on the “Look At” gesture, where the chairs orient themselves towards the viewer, and see how that interaction differs between chair arrangements. “Look At” is fundamentally the “Tracking You” gesture from the Agent Gestures study, which we have found to have scored high on responsiveness and relationship to audiences. We now evaluate this particular gesture with different initial chair arrangements.
Do viewers find the tracking gaze of the chairs directed at them more pronounced when the chairs are initially arranged in a circle or in an aligned grid? I hypothesize that in the aligned configuration, people will focus attention mostly at the back of the room where the chairs are pointing, and thus will be more affected by the switch to the “Look at” gesture where the chairs point towards the viewer. I created two scenes that arrange the chairs 1. aligned towards the sliding doors behind where the viewer starts in VR, and 2. around a circle about the center of the room near where the viewer is (Figure 12).
Intermittently during the experience, the chairs will switch to “Look At” orientation and continuously focus the front of its body towards the viewer, at which point she will be surprised for the first time (Figure 13). I tested 9 university students from Parsons School of Design and Cornell Tech in each condition. I allowed subjects to move around in the environment for two minutes to get them acquainted before making the first “Look At” maneuver at randomized intervals, triggered from the joystick held by the experimenter. I allow them to experience the environment further with periodic “Look At” interruptions for approximately 10 minutes, then surveying their experience with questions like “how stunned are you when the chairs looked at you,” and “what purpose do you think the room serves.” The statement-based answers are coded for the qualitative analysis.
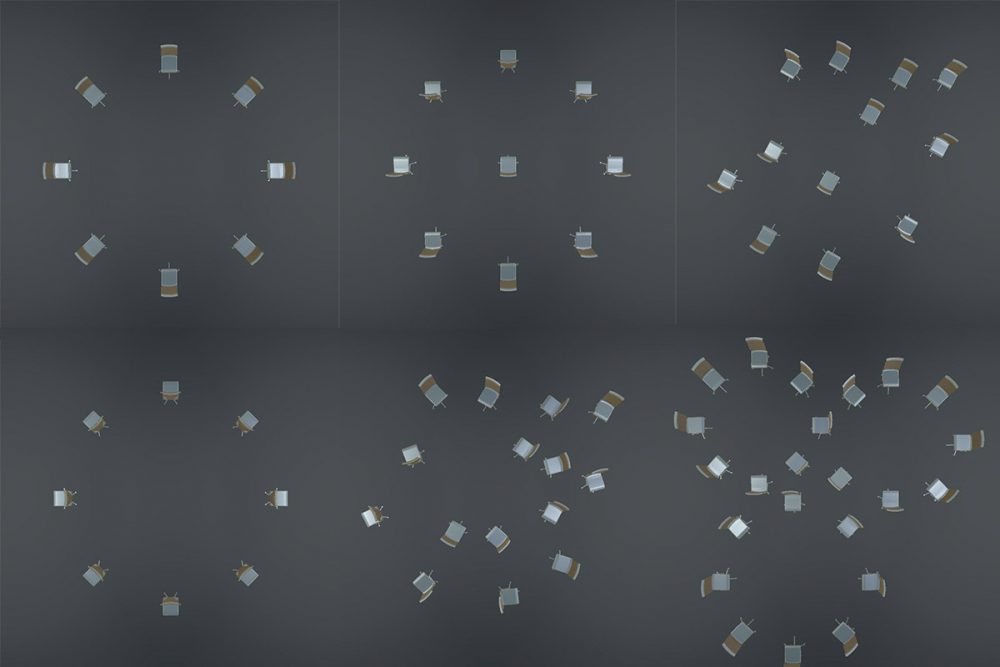
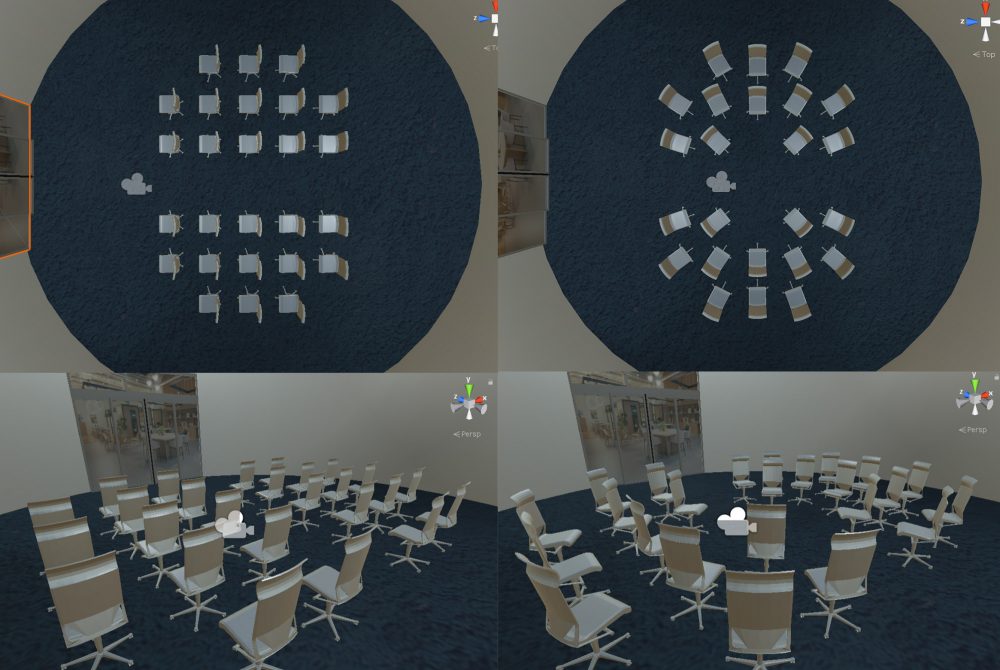
Results Summary
Ratings of the viewer to each question of “how expressive is the chair,” “how responsive is the chair,” and “how stunned are you when the chairs looked at you” are analyzed along with the configuration condition (aligned vs. circular) in a two-way ANOVA. The only significance found was in the configuration (p = 0.0426, Figure 14), and post-hoc test (Tukey HSD) also found only significance in aligned vs. circular conditions. Moreover the percentage of variance (eta squared) explained by configuration was 0.077, whereas the eta squared of question was 0.036, and eta squared of configuration:question interation was 0.030.
This shows that audiences are more stunned by the “Look At” tracking gesture employed by the chairs when they are initially in an aligned position towards the door rather than in a circular position around the center of the room. In the qualitative analysis, viewers assigned the room such functions as “meeting room” or “place of lecture” when given the aligned condition, consistent with the idea that aligned chairs provides a connotation of the room being used for events like talks and movie screenings. In the circular condition, people reacted that “chairs seem to be barriers,” and wonder if the room is “a waiting area” or “a place of discussion,” consistent with the idea of a circular arrangement for accentuation of the communal aspect of the environment.
Participants are absorbed by the setting and realism of the interaction in VR. One person even tried to sit on a virtual chair when it stopped moving, because it was designed to be life-sized with appropriate mechanics for control. The analysis shows that we can model interactions with audiences effectively in 3D in VR, providing a method to test spatial designs together with machine gesture interactions.
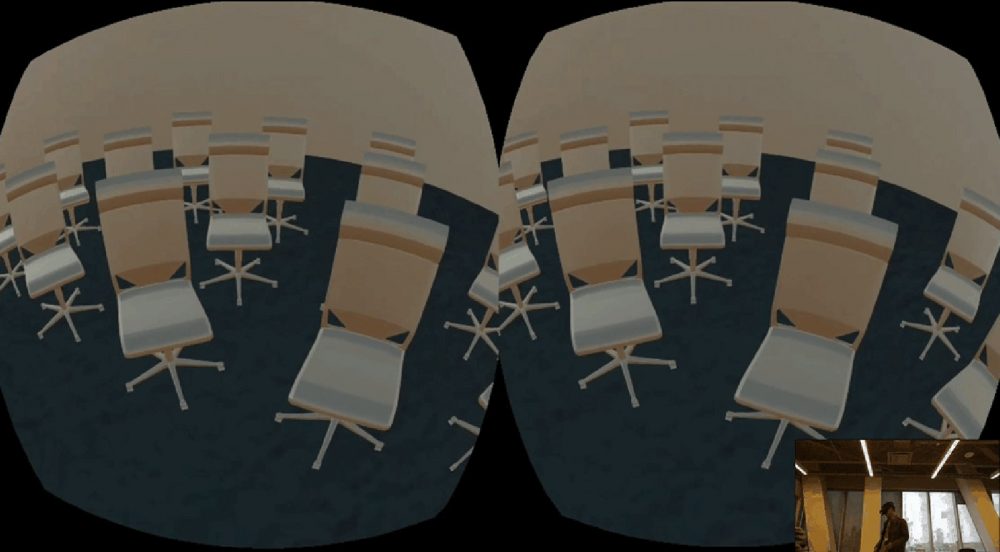
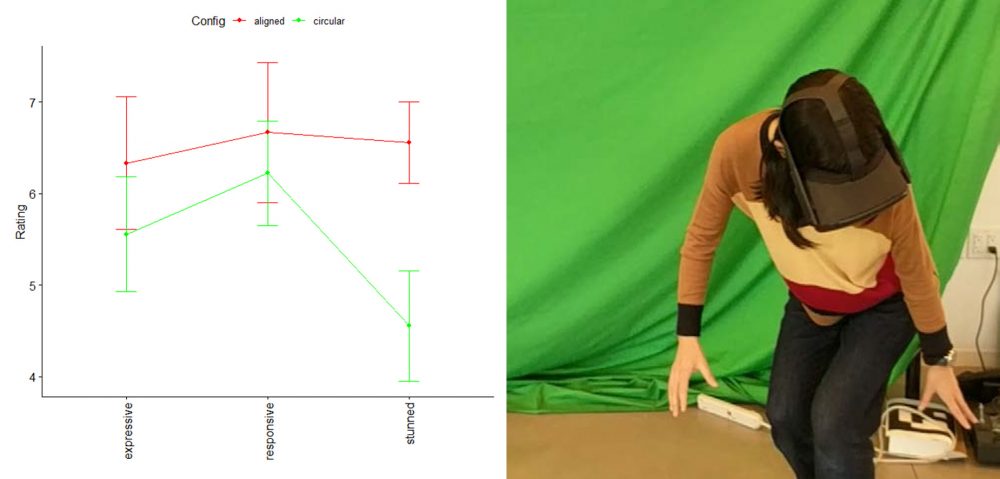
Discussion
Modeling interactions in VR has the advantage of not only showing difficult-to-realize prototypes, but also showing how such prototypes can interact with us. As this study shows, it also allows us to study situations that involve different spatial designs and impossible to choreograph interactions. The intricacies of physical prototypes that follow our faces exactly is difficult to execute, and in VR we have the opportunity to make as many of these prototypes and interactions as we desire, scaling up to monumental levels (like thousands of chairs in an auditorium) if we so choose.
In addition to testing spatial arrangements and how gestures can affect our perception in different arrangements, we can also study how locomotion affects our response. I have prototyped an 3D environment consisting of multiple moving chairs that follow paths in 3D, look at each other’s movements, or stop and go along some choreographed paths (Figure 15). These environments allow us to propose hypothetical questions about virtual prototypes that would be difficult to realize in practice. For example, I can put moving chairs in the environment and see what speed of interaction captures optimal attention, and then look at how different gestures are perceived when the locomotion is paused at different speeds.
Prototyping interactions between humans and robots in VR has been attempted in the arena of manufacturing, where precise control is needed (Matsas, Vosniakos, and Batras 2018). However, VR prototyping for interactions in spatial designs are now opened to us because headsets no longer need to be fixed down in space (much like the Oculus Quest I am using). Unlike the video prototypes, in VR we can model their interactions with respect to us as well, allowing us to test hypotheses regarding their interactions with us as I have shown in the space arrangement and “Look At” gesture study. Whereas in the videos we can only examine one interaction at a time in particular contexts, in VR we can put in as many interactions as desired based on different rules of gestural interaction. Moreover we can put these gestures in any number of agents as we like arranged in space the way we want to maximally affect attention and perception. Unlike the space design study, in VR we can examine gestural efficacy through activity in the objects themselves, rather than passively showing audiences images that correspond to snapshots in time. The VR prototype can both evaluate chair arrangements that affect audience response, and trigger machine gestures that occur in particular arrangements and see how audiences interact with them. This is useful for learning how to design in space that makes certain robotic gestures effective, since like in real-life, arrangements in VR can be changed without audiences looking.
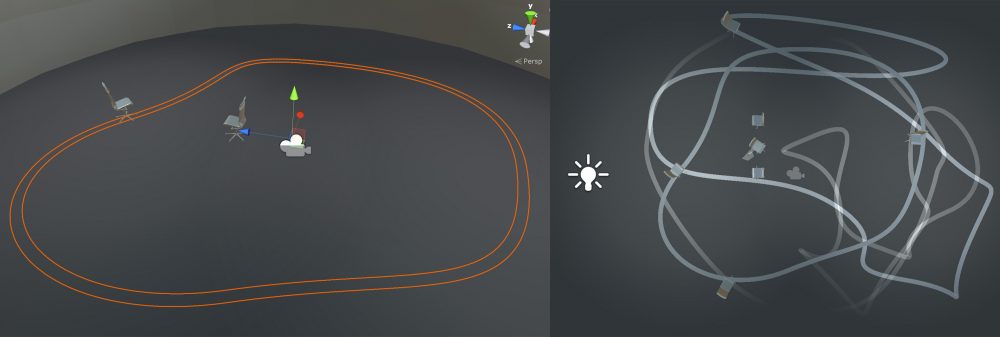
CHAPTER VIII: CONCLUSIONS
I have outlined a VR experience that uses both arrangement of chairs in space and gestural interactions of chairs to influence player perception. By choreographing a consistent reaction that depends on where the user is looking and moving towards in different spatial arrangements, we can begin to understand how people react to robotic gestures and the space that robots mark out, by creating interactions that cannot be easily reproduced in physical reality.
Together these studies in spatial influence, agent gestures, and 3D interactive immersive prototypes show the capability of machines to affect human perception and behavior both in the way it is arranged in space and in the interactions they maintain with us. They foretell a future where interactive furniture embedded in environments can shape our attention and response psychologically for the better by using spatial and gestural cues in concert to optimally alter human capabilities.
ACKNOWLEDGMENTS
I would like to thank Jess Irish, Danielle Jackson, Sven Travis, and Loretta Wolozin (Parsons School of Design); Wendy Ju, Natalie Friedman, and J.D. Zamfirescu-Pereira (Cornell Tech); and Judith Hall (Northeastern University) for their support, mentorship, and collaboration.
REFERENCES
Adams, Alexander T., Jean Costa, Malte F. Jung, and Tanzeem Choudhury. 2015. “Mindless Computing: Designing Technologies to Subtly Influence Behavior.” In Proceedings of the 2015 ACM International Joint Conference on Pervasive and Ubiquitous Computing, 719–730. UbiComp ’15. New York, NY, USA: ACM. https://doi.org/10.1145/2750858.2805843.
Breazeal, Cynthia, Nick DePalma, Jeff Orkin, Sonia Chernova, and Malte Jung. 2013. “Crowdsourcing Human-Robot Interaction: New Methods and System Evaluation in a Public Environment.” J. Hum.-Robot Interact. 2 (1): 82–111. https://doi.org/10.5898/JHRI.2.1.Breazeal.
Douglas, Darren, and Robert Gifford. 2001. “Evaluation of the Physical Classroom by Students and Professors: A Lens Model Approach.” Educational Research 43: 295–309. https://doi.org/10.1080/00131880110081053.
Drew, Clifford J. 1971. “Research on the Psychological-Behavioral Effects of the Physical Environment,.” Review of Educational Research 41 (5): 447–65. https://doi.org/10.3102/00346543041005447.
Görür, Orhan, Benjamin Rosman, Guy Hoffman, and Sahin Albayrak. 2017. “Toward Integrating Theory of Mind into Adaptive Decision- Making of Social Robots to Understand Human Intention.” In .
Ju, Wendy. 2015. The Design of Implicit Interactions. 1st ed. Morgan & Claypool Publishers.
Jung, Malte, and Pamela Hinds. 2018. “Robots in the Wild: A Time for More Robust Theories of Human-Robot Interaction.” ACM Trans. Hum.-Robot Interact. 7 (1): 2:1–2:5. https://doi.org/10.1145/3208975.
Kaye, Stuart M., and Michael A. Murray. 1982. “Evaluations of an Architectural Space as a Function of Variations in Furniture Arrangement, Furniture Density, and Windows.” Human Factors 24 (5): 609–18. https://doi.org/10.1177/001872088202400511.
Knight, Heather, Timothy Lee, Brittany Hallawell, and Wendy Ju. 2017. “I Get It Already! The Influence of ChairBot Motion Gestures on Bystander Response.” 2017 26th IEEE International Symposium on Robot and Human Interactive Communication (RO-MAN), 443–48.
LC, RAY. 2019. “Secret Lives of Machines.” Proceedings of the IEEE ICRA-X Robotic Art Program, Expressive Motions, Elektra, Montreal, Canada, no. 1: 23–25. Online: http://roboticart.org/wp-content/uploads/2019/05/05_RayLC_ICRARoboticArt2019_03.pdf
Luo, Pengcheng, Victor Ng-Thow-Hing, and Michael Neff. 2013. “An Examination of Whether People Prefer Agents Whose Gestures Mimic Their Own.” In Intelligent Virtual Agents, edited by Ruth Aylett, Brigitte Krenn, Catherine Pelachaud, and Hiroshi Shimodaira, 229–238. Berlin, Heidelberg: Springer Berlin Heidelberg.
Matsas, Elias, George-Christopher Vosniakos, and Dimitris Batras. 2018. “Prototyping Proactive and Adaptive Techniques for Human-Robot Collaboration in Manufacturing Using Virtual Reality.” Robotics and Computer-Integrated Manufacturing 50 (April): 168–80. https://doi.org/10.1016/j.rcim.2017.09.005.
Mol, Lisette, Emiel Krahmer, Alfons Maes, and Marc Swerts. 2007. “The Communicative Import of Gestures: Evidence from a Comparative Analysis of Human-Human and Human-Machine Interactions.” In AVSP.
Roberts, Adam, Hui YAP, Kian KWOK, Josip Car, Chee-Kiong Soh, George Christopoulos, and S. Chee-Kiong. 2019. “The Cubicle Deconstructed: Simple Visual Enclosure Improves Perseverance.” Journal of Environmental Psychology 63. https://doi.org/10.1016/j.jenvp.2019.04.002.
Sirkin, David, Brian Mok, Stephen Yang, and Wendy Ju. 2015. “Mechanical Ottoman: How Robotic Furniture Offers and Withdraws Support.” In Proceedings of the Tenth Annual ACM/IEEE International Conference on Human-Robot Interaction, 11–18. HRI ’15. New York, NY, USA: ACM. https://doi.org/10.1145/2696454.2696461.
Sommer, Robert. 1959. “Studies in Personal Space.” Sociometry 22 (3): 247–260.
Taher, Randah. 2008. Organizational Creativity through Space Design. Buffalo, NY: Buffalo State College.
Thomaz, Andrea, Guy Hoffman, and Maya Cakmak. 2016. “Computational Human-Robot Interaction.” Foundations and Trends® in Robotics 4 (2–3): 105–223. https://doi.org/10.1561/2300000049.
Wardono, Prabu, Haruo Hibino, and Shinichi Koyama. 2012. “Effects of Interior Colors, Lighting and Decors on Perceived Sociability, Emotion and Behavior Related to Social Dining.” Procedia – Social and Behavioral Sciences 38: 362–372. https://doi.org/10.1016/j.sbspro.2012.03.358.
Warner, Scott, and Kerri Myers. 2010. “The Creative Classroom: The Role of Space and Place toward Facilitating Creativity.” Technology Teacher 69.
Weinstein, Carol S. 1977. “Modifying Student Behavior in an Open Classroom Through Changes in the Physical Design.” American Educational Research Journal 14 (3): 249–62. https://doi.org/10.3102/00028312014003249.
Zhu, Rui, and Jennifer J. Argo. 2013. “Exploring the Impact of Various Shaped Seating Arrangements on Persuasion.” Journal of Consumer Research 40 (2): 336–49. https://doi.org/10.1086/670392.